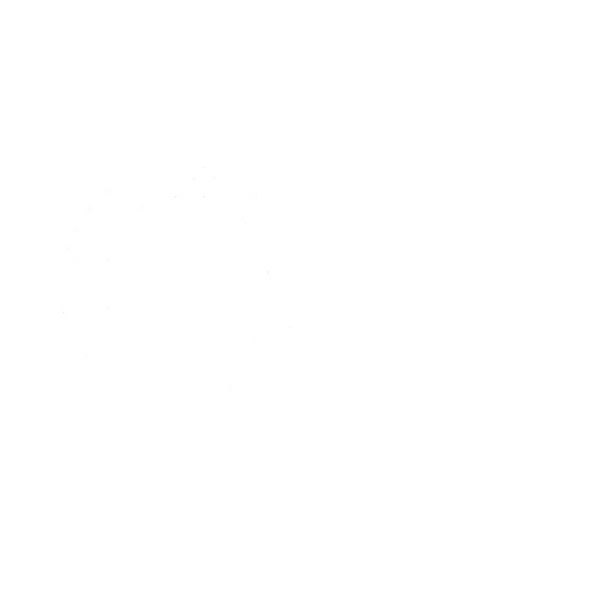
Welcome to MarchionniLab
Welcome to MarchionniLab
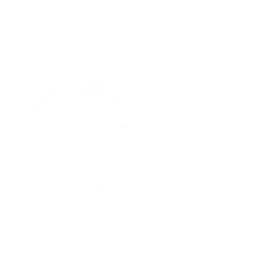
I am an Associate Professor of Pathology and Precision Medicine at the Weill Cornell Medical College in New York, USA. I am a doctor and a computational biologist working on cancer. I apply quantitative approaches to interpret high-dimensional genomic data, understand cancer cell biology, and develop useful clinical tools to improve cancer patients' outcomes.
My lab aims to improve human health, disease outcomes, and treatments through the development and application of innovative methods to analyze molecular and imaging data.
I am also the Vice-Chair for Computational and System Pathology.
Follow my lab on Twitter
Bioinformatics can be regarded as the field of intersection between biology and computer science. It uses the power of computation to store, analyze, integrate, and visualize complex biological data to answer biological questions. This field is concerned with organizing biomolecular databases, managing the quality of data input, getting useful information out of such databases, and integrating information from disparate sources. Genomics, systems biology, and high-throughput biological research are based on such sophisticated computer-based tools and advances in these fields would not have been impossible without the parallel development of Bioinformatics.
Millions of cells are dividing every minute in our human body. Normally, this process is well coordinated and cell divisions are followed by ordered patterning and cell specialization, allowing the maintenance of tissues and organs internal equilibrium (homeostasis). Cancer can be regarded as a deviation from this balanced behavior: a single cell, that has undergone mutations in its DNA, instead of maturing and dying normally reproduces without restraint. This is usually accomplished by incessant rather than faster dividing and it gives rise to a progeny that usually fails to mature. In this perspective it is clear that cancer is a very complex disease, involving not only cancerous cells, but also surrounding and distant normal cells, which engage an infinite number of complex interactions. Thus the effort of unraveling such a complexity by using genomic approaches is the focus of my laboratory.
Technological advances over the past decades have enabled the development of tools, systems, and infrastructure for the massive and parallel digitization of pathology slides with the associated meta‐data, their storage, review, and analysis. At the same time, advances in algorithmics, statistics, mathematics, and computer science have provided the tools for the extraction and analysis of quantifiable information from these images. This has created unprecedented opportunities for the systematic and quantitative analysis of images routinely generated in pathology departments around the world. The adoption of Artificial Intelligence (AI), Machine Learning (ML) – including more data and computation intensive approaches like Deep Learning (DL) – holds the promise to project pathology in the next millennium.
Medicine is transitioning from treating the “average patient” to seeking to treat each individual in a tailored way. At present, this approach to medicine, however, can only be delivered at lead academic institutions, hence the opportunity to deliver state of the art clinical care on a large scale and in the community is still missed. By integrating big molecular data, standard clinical laboratory measurements, and digital pathology images, my laboratory strive to bridge this gap. The goal here is to develop robust, parsimonious models that can forecast patients molecular make-up (e.g., their mutational profiles) and outcome (e.g., their response to targeted treatments). While the development of these approaches can only be achieved in leading academic institutions, deploying and disseminating such methods in community and rural hospitals nationwide is of paramount importance. Ultimately, through research in this domain, my lab fosters the democratization of precision and personalized medicine.
For the complete list of publications see
my google scholar or my my NCBI publication list
Eddie Luidy Imada
Mohammed Omar
Wikum Dinalankara
Claudio Zanettini
Karen Zhuoran Xu
Lucio Queiroz
Classes, workshops, lectures, and resources are listed here
Associate Professor of Pathology and Laboratory Medicine
Vice Chair for Computational and Systems Pathology
Weill-Cornell Medicine
413 East 69th Street,
The Belfer Research Building, Suite 1524
New York, NY, 10021, USA
Tel: (001) 646-962-8767
Fax: (001) 646-962-0437
Copyright © 2023 MarchionniLab - All Rights Reserved.
Powered by GoDaddy
We use cookies to analyze website traffic and optimize your website experience. By accepting our use of cookies, your data will be aggregated with all other user data.